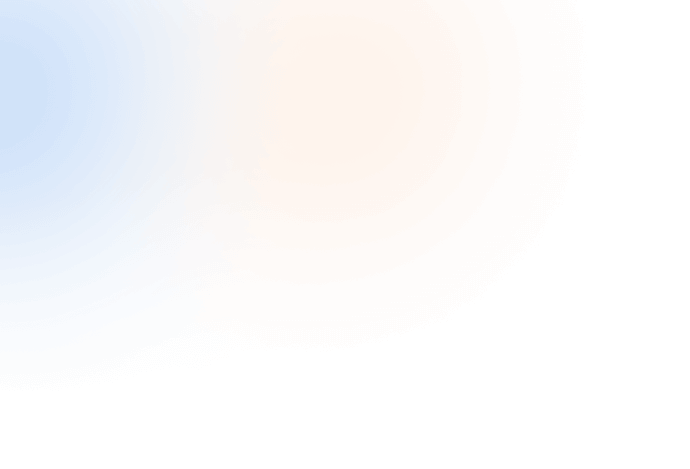
Convolutional Neural Networks: Revolutionizing Image Recognition in Business
- Home
- Article
29th October 2024
Share this Article
Convolutional Neural Networks: Revolutionizing Image Recognition in Business
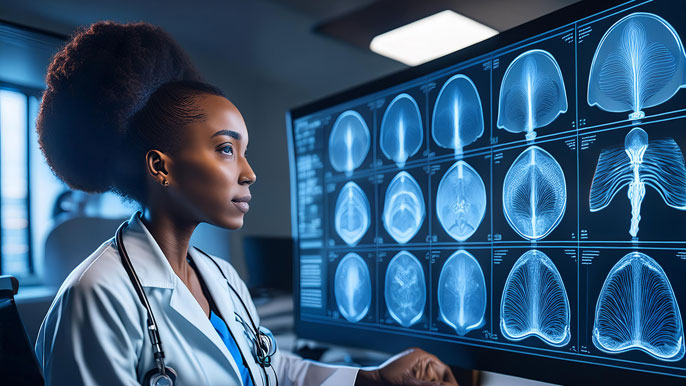
Convolutional Neural Networks (CNNs) are a specialized type of deep learning model, designed to process and interpret visual data. CNNs have gained immense popularity in recent years, thanks to their powerful capabilities in image and pattern recognition, making them indispensable across industries. Businesses in sectors like retail, healthcare, automotive, and security are leveraging CNNs to automate processes, enhance customer experiences, and make data-driven decisions. This article explores what CNNs are, how they work, and their transformative impact on business.
What is a Convolutional Neural Network (CNN)?
A Convolutional Neural Network is a deep learning algorithm that mimics the human visual system. Unlike traditional neural networks, CNNs are particularly effective in analyzing visual imagery due to their unique architecture. CNNs consist of multiple layers—convolutional layers, pooling layers, and fully connected layers—that work together to detect and classify images. These layers allow CNNs to automatically and accurately recognize patterns, making them ideal for tasks like image classification, object detection, and facial recognition.
How CNNs Work
- Convolutional Layers
The convolutional layers are the core building blocks of a CNN. In these layers, the model scans the image by applying a set of filters to identify specific features like edges, textures, and colors. The filters, or "kernels," slide over the image, creating feature maps that highlight the presence of certain attributes. - Pooling Layers
Pooling layers help reduce the spatial dimensions of the feature maps, preserving essential features while reducing the computational load. This process, known as downsampling, makes the network more efficient and prevents overfitting by ignoring less critical information. - Fully Connected Layers
After the feature extraction process, fully connected layers interpret the features to classify the image. These layers are similar to traditional neural networks, where each neuron is connected to every neuron in the previous layer, enabling the network to make predictions based on the detected patterns.
Applications of CNNs in Business
- Retail and E-commerce
CNNs play a significant role in product recommendation engines, visual search, and customer behavior analysis. Retailers can use CNNs to recommend products based on visual similarity, allowing customers to find items that match their preferences. Visual search, which lets customers search by images, enhances the shopping experience and drives sales. - Healthcare
In healthcare, CNNs assist in diagnosing medical conditions through image analysis. For example, CNNs can analyze X-rays, MRI scans, and CT scans to identify diseases, tumors, or fractures, providing doctors with accurate and timely insights. This technology not only improves diagnostic accuracy but also helps in remote healthcare applications. - Automotive
CNNs are fundamental to autonomous driving technology. They enable self-driving cars to detect and classify objects on the road, such as pedestrians, traffic signs, and other vehicles. This capability is crucial for navigating complex environments and making real-time decisions, improving the safety and efficiency of autonomous vehicles. - Security and Surveillance
CNNs enhance security by enabling facial recognition, object detection, and anomaly detection. Companies use CNN-powered systems to monitor security footage, identify unauthorized individuals, and even detect suspicious behavior. This technology is widely used in airports, shopping malls, and other public spaces to enhance safety. - Financial Services
In finance, CNNs are used to detect patterns in data, such as analyzing handwriting on checks, scanning financial documents, or identifying fraud through visual patterns. Financial institutions benefit from this technology by speeding up verification processes and reducing manual errors.
Benefits of CNNs in Business
- Automation and Efficiency
CNNs automate processes that would otherwise require manual labor, saving time and reducing costs. This is particularly useful in industries where visual data processing is essential, such as healthcare diagnostics and retail analytics. - Enhanced Accuracy
CNNs provide high accuracy in image and pattern recognition, improving decision-making. For example, in healthcare, CNNs reduce the risk of diagnostic errors, while in retail, they help identify product similarities for better recommendations. - Data-Driven Insights
By analyzing visual data, CNNs provide valuable insights into customer behavior, market trends, and operational efficiency. Businesses can use this information to refine their strategies and improve customer satisfaction. - Scalability
CNNs are highly scalable, allowing businesses to process large volumes of visual data efficiently. As businesses grow and accumulate more data, CNNs can handle the increased load without compromising performance.
Understanding Biases in Machine Learning
While CNNs are revolutionizing industries, it's essential to remain cautious of biases in machine learning. Just as the narrative fallacy can skew investment decisions, biases in training data can affect the outcomes of CNNs. If you're interested in learning more about cognitive biases in business, check out our article on The Narrative Fallacy in Investing: How Stories Influence Financial Decisions.
CNNs as a Game-Changer for Visual Data Processing
Convolutional Neural Networks have opened new horizons for businesses by transforming how they handle visual data. From enhancing customer experiences in retail to assisting in critical medical diagnoses, CNNs have become indispensable across industries. As this technology continues to evolve, businesses that leverage CNNs will gain a significant edge in efficiency, accuracy, and scalability.
With their remarkable ability to process and interpret visual data, CNNs are shaping the future of business, making operations smarter, faster, and more insightful.
Start the conversation
Become a member of Bizinp to start commenting.
Already a member?
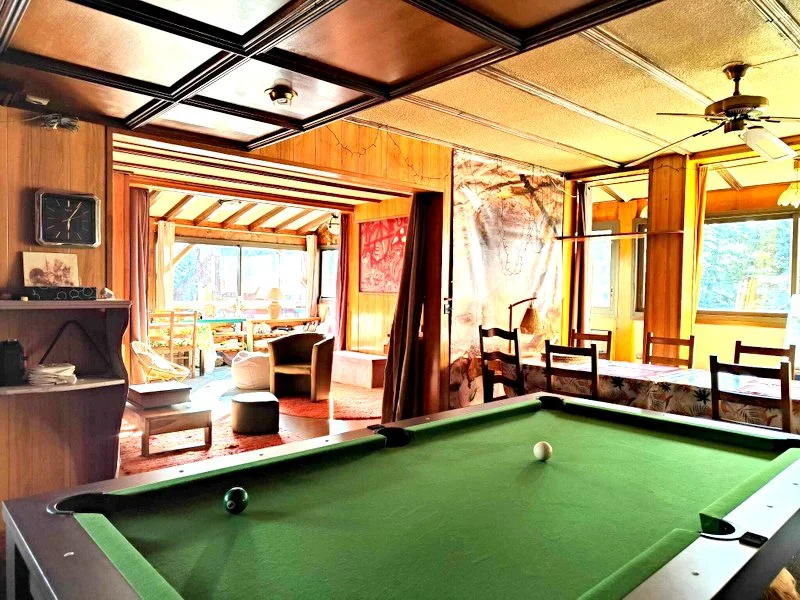
Small restaurant for sale in French Ski Resort
Great opportunity for people wishing to move to France on an entrepreneur visa. This small café/restaurant is located in the centre of one of France's most attractive ski resorts in the Pyrenees. It includes space for 40 diners, a fully-equipped professional kitchen and accommodation for the owner/manager. The very attractive price of €160,000 includes all the furniture and equipment as well as the agency fee: the business is ready to roll! More info directly from us at [email protected] There are two main seasons - Christmas through to end March (winter sports) and summer season from mid-June to the end of October (hiking, walking, nature clientele).